Insurance people used to think of pricing as just a market thing – nothing to do with ethics or misconduct. Now they recognise that fairness has to be factored in as well. Yet the ethics of insurance pricing has other dimensions that both the regulator and market need to tune in to. If they don’t, the current pricing review will take even longer and become even more tortuous.
The regulator often urges insurers to focus more on outcomes. In essence, look not just at what you do, but on the outcomes that that produces as well. This is fine, so long as what you think of as outcomes makes sense. The FCA’s interim pricing report was so margins based that it begged the obvious question: is this what they see as outcomes?
If it is, then another question then rears its head: have they understood the super-complaint in the same way as the people who submitted it? To illustrate what I mean here about outcomes, I’m going to go back to 2015 and an FCA field study report on household insurance pricing that signalled to me that the regulator was up to something. A key graph in the report presented this data:
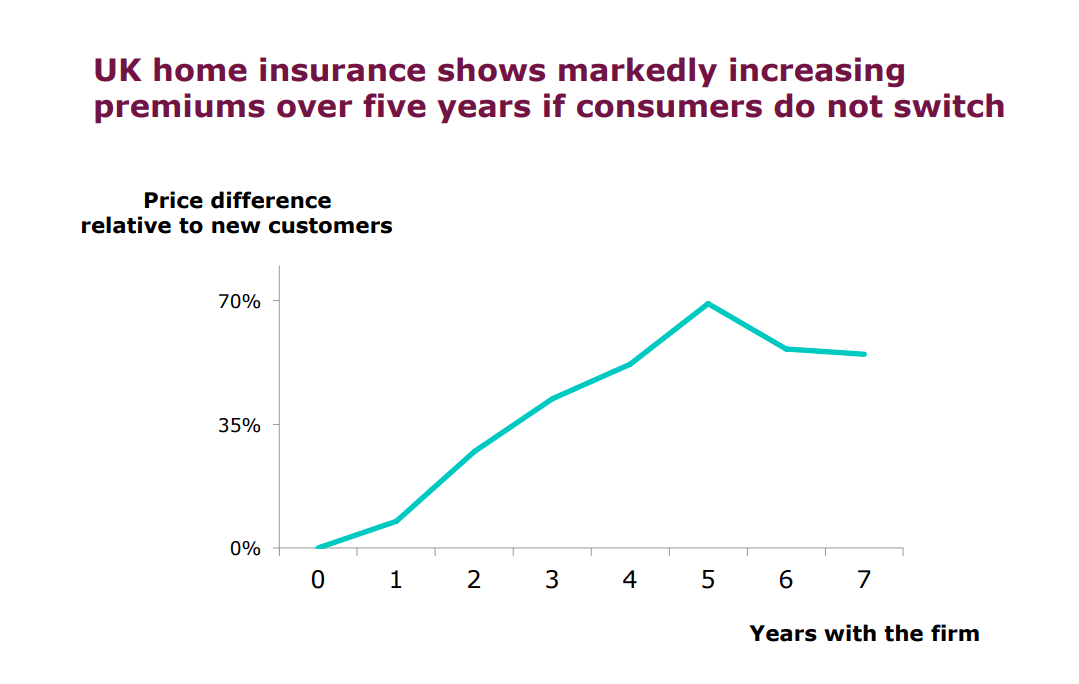
Problem? What Problem?
Yet, ten months later, the FCA published a report on big data, in which one of the standout conclusions was that they saw little problem with the personalisation trend in insurance pricing. And that message was reiterated to me a few years ago by one of the 2015 field study authors. Personalisation was just one of those market trends.
Move on to early 2018 and two blog posts I wrote, warning the market that an ethical storm was brewing around its price practices. In the second of those two posts, I included the above graph. Not long afterwards, I was contacted by both BBC Radio and a leading newspaper, wanting to know more about the issues in the post, and in particular the graph. To them, the graph signalled all sorts of red warning lights.
How then can one graph speak differently to different audiences? After all, aren’t graphs just neutral visuals, full of objective numbers? Well, this particular graph sent out mixed signals. It presented outputs of an economic analysis of pricing outcomes. What it didn’t present was what those outcomes represented. The significance of that difference became obvious when Citizens Advice submitted their super-complaint.
However, what the news media still only saw in that graph was economic analysis, this time interpreted with a more independent and critical eye perhaps than insurers or the regulator. What few saw in that graph were the experiences that Citizens Advice had been seeing, and which motivated them to issue the super-complaint.
Data and Graphs are not Neutral
Now some of you will question the significance of this. It’s just a graph after all. Yet I would say it is significant because the purpose for which one gathers, analyses and presents data will influence each of those stages. I’ll illustrate this through that same graph.
What the graph told us was that the field study found on average that people were paying at yearly intervals, various percentages more for their cover than when they started out as new customers. The standout pricing data point was 70% more at 5 years. What the graph did not show however was what those percentages actually meant in real life.
For example, it doesn’t make clear the cumulative impact of all those extra premiums being paid. So in year 1, I paid say 10% more, in year 2 30% more and so on. I may have been paying 70% more by year 5, but that is still only a snapshot. What I’ve experienced is the cumulative loss of money. Taken together, this amounts to much more than any introductory offer in year one.
Judging Significance
Let’s take the obvious next step with this. Those annual percentages (as in 70% after 5 years) may look quite large, but the data scientist, underwriter or regulatory manager will know that because the average household premium upon which they’re premised is a few hundred pounds, the actual pounds amount of those percentages will feel, to them, not that significant. Not good of course, but not significant.
Yet if you look at the amount on an accumulated basis, and look at it from the perspective of say, a pensioner, both the accumulated amount feels very significant, and what it represents even more significant. To a pensioner, that accumulated amount represents several months of pension. It represents the loss of opportunities to heat their home a little bit more over winter, to treat themselves to something nice from time to time, to do things that add comfort into their lives.
Let’s see this from another perspective. The busy single parent who just auto renewed their policy for several years, and paid more over those years as a result, lost opportunities around childcare, around something new for the kids, around treats or time off for themselves.
What these people, and many more like them, did not experience was 70% at 5 years. Instead, they experienced outcomes that directly affected life choices they had to make.
Making Data Meaningful
So what can be done differently, you may ask?
It would be quite straightforward to take that ‘70% at 5 years’ graph and change it, to show representations of those loss opportunities. For example, how many months pension does that accumulated extra premium spend on average add up to? How many hours of average childcare cost?
Such measures are of course more emotive, and some people, in both the regulator and the market, will say that emotion should not get involved in how products like insurance are judged. That’s fine if you’re well off and see and judge the market through technical and economic lens. It’s not fine if you’re hard up and see the market through the lens of tight budgets and those micro-financial / micro emotional crisis I’ve written about before.
Now some of you may think something along the lines of “I will choose my own lens thank you very much”. And I understand that, but at the same time, the lens you do choose must reflect the responsibilities you have, the accountabilities you have. And some of those accountabilities will not be of your own choosing. The super-complaint made that very clear.
If in the 2015 field study, if in the 2016 big data report, if in the period up to October 2018, someone had turned the ‘70% at 5 years’ graph around, and shown it instead in terms of, for example, the number of pension months lost, then more people would have understood the pricing problem much earlier. And I say that in respect of both the market and the regulator.
Better Conversations
Can such small changes make that much of a difference? Well, I think they can, for they demonstrate that you’re seeing your data as more than just numbers, as more than just economic trends. How you see your data signals to others equally interested in that data, that you’re on a similar wavelength, that you recognise their concerns, that you have been listening. This facilitates better conversations, better understandings, better relationships.
This is not just a regulatory issue. Insurers themselves should be producing more meaningful management information around their pricing strategies. All too often, it is just a wall of economic statistics. The customer experience is hard to find. Is this a terribly complicated thing to do though? Not at all. Insurers using the lifetime value model have all the granularity of data they need.
What will motivate them to do so? I think this will emerge out of the debate that the publication of the FCA’s ‘final’ pricing report will throw up. I fear that that report will go some way but ultimately fail to satisfy Citizens Advice’s concerns. Like the interim report, it will lack resonance with how the organisation thinks, with what their people on the ground have been experiencing.
Warning Signs
I’m already hearing of such failed conversations in other quarters relating to insurance. Yes, there are successful joint initiatives involving the sector and civil society groups, but I’m also hearing of serious concerns as well. And what happens because of this is a disengagement from the sector and an engagement with a more political process. That’s why I mentioned earlier on, that we can’t always chose what we’re held accountable for.
I’m in the middle of some research into data and power, and this blog post has emerged from some initial findings, influenced by the data visualisation work of Periscopic. To end, I’m going to re-quote from an earlier blog post, something that Professor Terras of Edinburgh University’s Futures Institute said at a lecture at the Alan Turing Institute in 2019:
All data is historical data: the product of a time, place, political, economic, technical, & social climate. If you are not considering why your data exists, and other data sets don’t, you are doing data science wrong.”
Data science is going to transform insurance. What we need to be sure of, is that we’re doing our data science right, that it’s helping drive insurance towards the superhighway, and not off a cliff.